Nominal, Ordinal, Interval, Ratio Scales
Understanding Levels of Measurement in Statistics
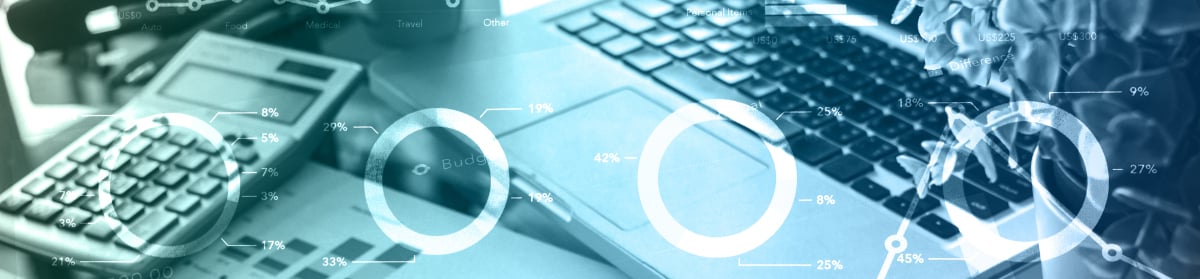
Understanding the levels of measurement is fundamental for researchers and statisticians as it dictates the type of statistical analysis that can be appropriately applied to the data. Measurement scales categorize the nature of variables and determine how they can be manipulated and interpreted. The four primary types of measurement scales are nominal, ordinal, interval, and ratio scales. Each scale has unique properties that influence data analysis and the conclusions drawn from research.
In statistical analysis, variables are classified based on their measurement scales, which can significantly impact the choice of analytical methods. For instance, a categorical variable like gender typically uses a nominal scale, whereas a variable like temperature uses an interval scale. Proper identification of whether a variable is dependent or independent also relies on understanding its measurement level. The distinction between these scales ensures that data is handled correctly, maintaining the integrity of the research findings.
This guide explores each level of measurement in detail, providing definitions, examples, and practical applications. By comprehensively understanding nominal, ordinal, interval, and ratio scales, researchers can make informed decisions about data collection, analysis, and interpretation, ultimately enhancing the quality and reliability of their studies.
Comparison of Measurement Scales
Scale | Type | Ordering | Arithmetic Operations | True Zero |
---|---|---|---|---|
Nominal | Categorical | No | No | No |
Ordinal | Categorical | Yes | No | No |
Interval | Quantitative | Yes | Yes | No (Arbitrary) |
Ratio | Quantitative | Yes | Yes | Yes |
Nominal Scale: Definition and Examples
A nominal scale is the most basic level of measurement in statistics. It categorizes data without any inherent order or ranking among the categories. Nominal scales are used for variables that represent discrete categories, where each category is mutually exclusive and exhaustive. This means that each data point can only belong to one category and that all possible categories are represented.
Nominal scales are primarily used for categorical variables, such as gender, race, or types of cuisine. In surveys and research, nominal scales help in organizing data into meaningful groups, allowing researchers to analyze the frequency and distribution of each category. For example, when conducting a survey on favorite types of transportation, responses might include categories like 'Car', 'Bicycle', 'Bus', and 'Train'.
Importantly, because nominal scales do not imply any order or magnitude, statistical operations are limited. Researchers can calculate mode and frequencies, but measures like mean or median are not applicable. Understanding nominal scales is essential for accurate data classification and appropriate statistical analysis.
Common Nominal Variables
Variable | Possible Values |
---|---|
Gender | Male, Female, Non-Binary, Prefer Not to Say |
Marital Status | Single, Married, Divorced, Widowed |
Education Level | Primary, Secondary, Tertiary, Post-Graduate |
Favorite Dessert | Ice-Cream, Pie, Pastries, Donuts |
Categorizing Nominal Variables
Nominal Variable | Categories |
---|---|
Favorite Beverage | Coffee, Tea, Soda, Water |
Type of Pet | Dog, Cat, Bird, Fish |
Mode of Transportation | Car, Bicycle, Bus, Train |
Country of Origin | USA, Canada, UK, Australia |
For further reading on nominal scales and categorical data, refer to the comprehensive resources available at the University of New South Wales and other authoritative sites listed in the Helpful References section.
Ordinal Scale: Understanding Ranking and Order
An ordinal scale provides a way to categorize data with a meaningful order or ranking among the categories. Unlike nominal scales, ordinal scales indicate the relative position of each category, but they do not specify the magnitude of differences between them. This means that while you can say one category is higher or lower than another, the exact difference is not quantifiable.
Ordinal data is commonly used in surveys and questionnaires where responses are ranked or ordered. For example, satisfaction levels in a survey might include categories like 'Very Unsatisfied', 'Unsatisfied', 'Neutral', 'Satisfied', and 'Very Satisfied'. Here, the order of responses matters, but the intervals between each level are not consistent or measurable.
In research, ordinal scales are useful for measuring attitudes, preferences, and perceptions where the focus is on the order rather than the precise value. However, statistical analysis with ordinal data is limited to non-parametric methods, as the exact differences between ranks are not known.
Example of Ordinal Data
Survey Question | Responses |
---|---|
Rate your satisfaction with our service: | 1 - Very Unsatisfied, 2 - Unsatisfied, 3 - Neutral, 4 - Satisfied, 5 - Very Satisfied |
Rank the following desserts from most to least favorite: | 1 - Ice-Cream, 2 - Brownies, 3 - Cheesecake, 4 - Cake, 5 - Pie, 6 - Donuts, 7 - Pastries |
For more detailed insights into ordinal scales and their applications, visit resources such as CUNY's Measurement Guide and other educational websites referenced in the Helpful References section.
Interval Scale: Measuring Differences with Equal Intervals
An interval scale is a level of measurement where the differences between values are meaningful and consistent, but there is no true zero point. This means that while you can measure the exact differences between measurements, you cannot make statements about how many times greater one value is compared to another.
Interval data allows for the calculation of statistical measures such as the mean, median, and standard deviation because the intervals between values are uniform. However, since there is no true zero, ratio comparisons (e.g., twice as much) are not possible. A classic example of an interval scale is temperature measured in Celsius or Fahrenheit. In these scales, zero does not represent the absence of temperature, and negative values are possible.
Interval scales are essential in fields like psychology and meteorology, where precise measurements of differences are required without needing to represent an absolute zero. They enable researchers to perform a variety of statistical analyses, enhancing the depth and accuracy of their findings.
Temperature as an Interval Scale
Temperature (°C) | Description |
---|---|
10°C | Cool |
20°C | Mild |
30°C | Hot |
Practical Example: Dessert Rating
Consider a scenario where participants rate desserts on a scale from 1 to 10. The ratings reflect the intensity of their preference, with equal intervals between each rating point. For instance:
- 10.0 - Brownies
- 9.0
- 8.5 - Pastries
- 8.0 - Ice-Cream
- 7.0
- 6.0 - Cheesecake
- 5.0 - Pie
- 4.0 - Cake
- 3.0
- 2.0
- 1.0 - Donuts
This rating system allows researchers to analyze the average preference and variability among participants. However, it's important to note that while the differences between ratings are consistent, the absence of a true zero means that the scale cannot express absolute quantities.
For more comprehensive information on interval scales and their applications, explore the resources provided by University of Michigan's Statistics Department and other authoritative references listed in the Helpful References section.
Ratio Scale: Absolute Measurement with a True Zero
The ratio scale is the highest level of measurement in statistics, characterized by the presence of a true zero point. This means that zero on a ratio scale represents the complete absence of the measured attribute, allowing for meaningful comparisons of absolute magnitudes. Unlike interval scales, ratio scales enable the calculation of ratios, making it possible to state that one value is twice or half another value.
Ratio data is essential in scientific research and fields that require precise and absolute measurements. Common examples include weight, height, duration, and income. Since ratio scales have all the properties of interval scales, including ordered categories and equal intervals, they also allow for a full range of statistical analyses, including geometric and harmonic means.
The true zero in ratio scales is crucial because it signifies the absence of the quantity being measured. This allows for a more comprehensive and accurate representation of data, facilitating complex analyses and comparisons. Researchers rely on ratio scales when absolute quantification is necessary for their studies.
Height and Weight as Ratio Variables
Variable | Possible Values | Significance of True Zero |
---|---|---|
Height (cm) | 0, 50, 100, 150, 200 | 0 cm means no height |
Weight (kg) | 0, 10, 20, 30, 40 | 0 kg means no weight |
Practical Example: Dessert Baking
Imagine a baker measuring how high different desserts rise during baking:
- 16cm - Cake
- 14cm - Cheesecake
- 12cm
- 10cm - Pastries
- 8cm
- 6cm - Donuts
- 4cm - Pie
- 2cm - Brownies
- 0cm - Ice-Cream
This example demonstrates the utility of ratio scales in providing absolute measurements. The true zero point (0cm) indicates that ice-cream does not rise, and the differences in height can be precisely compared and analyzed.
For further exploration of ratio scales and their applications, visit resources such as Portland State University's Lecture Notes and other authoritative sites referenced in the Helpful References section.
Key Differences Between Nominal, Ordinal, Interval, and Ratio Scales
Understanding the key differences between the four levels of measurement - nominal, ordinal, interval, and ratio scales - is essential for selecting the appropriate statistical methods for data analysis. Each scale has distinct characteristics that determine the types of operations and analyses that can be performed.
Nominal scales categorize data without any inherent order, making them suitable for categorical variables where the primary interest is in frequency and mode. In contrast, ordinal scales not only categorize data but also rank them in a meaningful order, although the intervals between ranks are not equal or known.
Moving to the quantitative side, interval scales provide ordered categories with equal intervals between values, allowing for meaningful addition and subtraction, but lacking a true zero point. Ratio scales, on the other hand, include all the properties of interval scales and add a true zero, enabling the full range of arithmetic operations, including multiplication and division.
Comprehensive Comparison of Measurement Scales
Scale | Type | Ordering | Arithmetic Operations | True Zero | Examples |
---|---|---|---|---|---|
Nominal | Categorical | No | No | No | Gender, Marital Status, Favorite Dessert |
Ordinal | Categorical | Yes | No | No | Satisfaction Ratings, Class Rankings |
Interval | Quantitative | Yes | Yes (Addition, Subtraction) | No (Arbitrary) | Temperature (°C, °F), IQ Scores |
Ratio | Quantitative | Yes | Yes (All Arithmetic Operations) | Yes | Height, Weight, Time, Income |
Properly distinguishing between these scales ensures that data is analyzed using appropriate statistical techniques, enhancing the validity and reliability of research outcomes. Misclassification of measurement scales can lead to incorrect analyses and misleading conclusions.
Common Mistakes When Choosing Measurement Scales
Selecting the appropriate measurement scale is crucial for accurate data analysis. However, researchers often make common mistakes that can compromise the validity of their findings. Understanding these pitfalls and knowing how to avoid them can significantly enhance the quality of research.
Do's and Don'ts of Selecting Measurement Scales
Do's | Don'ts |
---|---|
Carefully assess the nature of your data and research objectives before selecting a scale. | Don't assume all numerical data fits into a ratio scale without verifying the presence of a true zero. |
Use nominal scales for categorical variables where classification is the primary goal. | Don't apply arithmetic operations to nominal or ordinal data where they are not meaningful. |
Ensure ordinal scales are used when the order of categories is important, but intervals are not equal. | Don't ignore the potential for misclassification, which can lead to incorrect statistical analyses. |
Choose interval or ratio scales for quantitative data that require precise measurements and statistical analysis. | Don't treat interval scales as if they have a true zero, which can lead to invalid ratio comparisons. |
To avoid these common mistakes, researchers should thoroughly understand the properties of each measurement scale and apply them consistently throughout their studies. Consulting comprehensive resources and guidelines, such as those provided by Monash University, can further aid in making informed decisions about measurement scales.
Frequently Asked Questions (FAQs)
What are the scales of measurement for a dependent variable?
How do you determine the level of measurement for a dependent variable?
What is a nominal scale and how is it used?
How are ordinal scales different from nominal scales?
What is the main difference between interval and ratio scales?
Can nominal data be used for statistical analysis?
What are the common examples of nominal variables?
What scale of measurement is used for independent variables?
How do nominal scales differ from categorical variables?
Can arithmetic operations be performed on ordinal data?
How is an interval scale used in surveys?
What are examples of ratio variables?
What is the significance of a true zero in ratio scales?
Why can't nominal data be ordered?
Can interval scales have a zero value?
What is the best way to analyze ordinal data?
How do you calculate the mean for interval data?
Why is it important to know the level of measurement in statistics?
What are some common uses of ratio data in research?
Physical Measurements: Variables like height, weight, distance, and volume are measured on ratio scales, allowing for precise calculations and comparisons.
Economic Data: Income, expenditure, and investment amounts are ratio variables that enable meaningful financial analyses and economic modeling.
Time-Based Metrics: Duration, response time, and lifespan studies rely on ratio data to assess temporal aspects accurately.
Scientific Research: Measurements in fields like chemistry and physics, such as concentration levels and velocity, use ratio scales for accurate experimentation and analysis.
Healthcare: Biometrics like blood pressure, cholesterol levels, and body mass index (BMI) are measured on ratio scales to monitor health status and outcomes.
The true zero point of ratio data allows researchers to perform a wide range of statistical analyses, including geometric means, coefficients of variation, and regression models, making it invaluable for generating robust and meaningful insights.
How does the scale of measurement affect data analysis?
Nominal Scale: Being the simplest level, nominal data is limited to categorization and frequency analysis. Statistical methods include mode identification, chi-square tests, and contingency tables, which help in understanding the distribution and relationships between categorical variables.
Ordinal Scale: With inherent order, ordinal data allows for median and percentile calculations, as well as non-parametric tests like the Mann-Whitney U test or the Kruskal-Wallis test. These methods account for the ranked nature without assuming equal intervals.
Interval Scale: Interval data supports a wider range of statistical operations, including mean, standard deviation, correlation, and regression analyses. The equal intervals enable more precise measurements of central tendency and variability.
Ratio Scale: The most versatile scale, ratio data allows for all statistical techniques applicable to interval data, plus additional analyses like geometric means, coefficients of variation, and complex modeling. The true zero point facilitates meaningful ratio comparisons and multiplicative operations.
Selecting the correct scale ensures the validity of statistical results, prevents misuse of analytical methods, and enhances the accuracy of data interpretation. It also influences data visualization choices, such as appropriate graph types and scaling, further aiding in effective communication of findings.
Helpful References:
- Australian Bureau of Statistics (2021). Statistical Language - What are Variables? https://www.abs.gov.au/websitedbs/D3310114.nsf/home/statistical+language+-+what+are+variables
- University of New South Wales (2021). Types of Data & the Scales of Measurement. https://studyonline.unsw.edu.au/blog/types-of-data
- An Introduction to Principles of Research (2021). What is Research
- Portland State University. Lecture Notes on Measurement Scales. https://web.pdx.edu/~newsomj/pa551/lecture1.htm
- Monash University. Scaling in Research. https://users.monash.edu/~smarkham/resources/scaling.htm
- University of Michigan. Types of Data in Statistics. https://dept.stat.lsa.umich.edu/~kshedden/introds/topics/types_of_data/
- CUNY. Measurement Scales in Research. http://media.acc.qcc.cuny.edu/faculty/volchok/Measurement_Volchok/Measurement_Volchok5.html